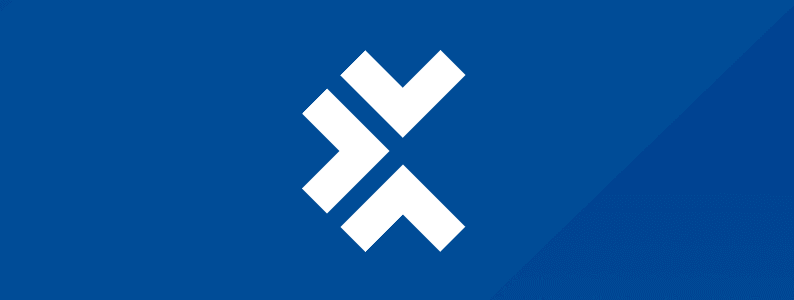
What is AI?
General AI (or true AI, strong AI) can be understood as a computer system with the ability to apply intelligence to any problem. That’s the holy grail of AI…
10 Examples of AI: From Sobering to Shocking
Stephen Hawking, Bill Gates, and Elon Musk have something in common, and it’s not wealth or intelligence (Paul Jongko). They are all terrified of the so-called AI apocalypse, a hypothetical scenario where a computer system possesses intelligence far surpassing that of the brightest human minds.
AI Approaches Compared: Rule-Based Testing vs. Learning
There are two main approaches to implementing AI in software testing: rule-based techniques and machine learning techniques.
The Business Impact of AI
AI is more an opportunity than a threat. Don’t see the difficulties; see the opportunities, accept the challenge, and take the chance…
How to test learning systems
The question that plagues most of us testers in the era of AI is, “Do we need to change our current test approaches to test learning systems?” In short, the simple answer is, “Yes, but only slightly!” Why is that?
AI-Driven Test Automation with Tosca
Tricentis Tosca, powered by Vision AI is our innovative solution to the rapid explosion of new applications and technologies enterprises are developing today, and the constant pressure this puts on Agile and DevOps teams to rapidly test these new technologies. With Vision AI, we have created the next generation of test automation – an AI-powered test execution technology that can track controls on any technology in real time, enabling automation at the speed of sight.
Watch “Vision AI: The 3rd Era of Test Automation”Watch “Vision AI: The 3rd Era of Test Automation”
More on AI-Driven Test AutomationMore on AI-Driven Test Automation
Testing AI Systems: Not as Different as You’d Think
AI-based tools have transformed from a vague, futuristic vision into actual products that are used to make real-life decisions. Still, for most people, the inner workings of deep-learning systems remain a mystery. If you don’t know what exactly is going on while the input data is fed through layer after layer of a neural network, how are you supposed to test the validity of the output? It’s not magic; it’s just testing.
The Great Debate: The Role of AI in Software Testing
AI in software testing is not a panacea that magically eliminates all your testing problems—but there are many ways that it can deliver business value today by helping you test smarter and more efficiently. Watch a lively panel discussion in which Wolfgang Platz (Tricentis Founder and Chief Strategy Officer), Tom Murphy (Senior Director Analyst), Jeff Wilkinson (Managing Director of Accenture), and other software industry leaders debate these and other AI-focused topics that are currently polarizing the software testing industry.
Beyond Continuous Testing with AI
Digital Transformation is forcing enterprises to innovate at lightning speed. While DevOps delivery cycle time is decreasing, the technical complexity required to deliver a positive user experience and maintain a competitive edge is increasing—as is the rate at which we need to introduce compelling innovations.
We’ve turned to Continuous Testing to bridge the gap in software test automation today, but how do we test when these trends continue and the gap widens tomorrow? We need “digital testing” to meet the quality needs of a future driven by IoT, robotics, and quantum computing. Artificial intelligence (AI) in software testing, imitating intelligent human behavior for machine learning and predictive analytics, can help us get there.
Learn how AI in software testing can take it to the next level, including:
- Why AI is now more feasible—and critical—than ever
- What AI really is and how it’s best applied
- How AI can help us test smarter, not harder