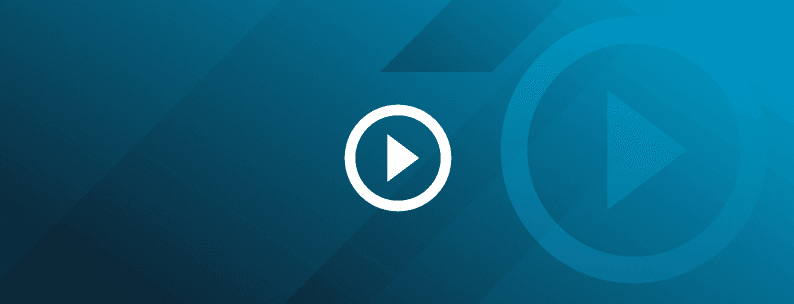
The role of data observability for enterprise data integrity
Data observability is a critical aspect of any data-driven business. It refers to the ability to monitor and troubleshoot data quality issues in real-time.
Data observability is a critical aspect of any data-driven business. It refers to the ability to monitor, understand, and troubleshoot data quality issues in real-time. In essence, data observability ensures that data is accurate, reliable, and accessible. It involves collecting and analyzing data from various sources to gain insight into how data is being used, how it is flowing through the system, and whether it is meeting the required quality standards.
Data integrity for evolving data ecosystems, cloud data warehouses, ETL integration pipelines, and BI reporting as well as AI/ML models is continually gaining importance as organizations attempt to transform the modern data explosion into insights and predictions that improve the customer experience and provide an edge against competition.
These factors, along with recent macroeconomic shifts, are major drivers for IT modernization and digital transformation initiatives. However, data quality issues at various stages throughout the enterprise data environment are a major challenge to the rapid development and implementation of data integration and management solutions. Many researchers have contributed to an understanding of data quality problems, collectively identifying general causes during these steps of data integration planning and execution.
Data observability: A key component for successful data-driven enterprises
Data observability is a new approach for data engineers, data architects, data officers and platform engineering teams to solve critical use cases that existing tools fail to address. As per Gartner research, “Data observability has now become essential to support as well as augment existing and modern data management architectures.”
Data quality is one of the most important components of data observability. Poor data quality can result in inaccurate insights, missed opportunities, and bad business decisions. Data quality issues can arise from various sources, including human error, system failures, data integration issues, and data transformation errors. Data observability allows businesses and especially Data Ops teams to monitor data quality in real-time, identify issues early, and take corrective action before they escalate.
What’s the difference between data observability and data integrity?
Data observability is becoming increasingly important in today’s data-driven business landscape. As organizations rely more on data to make decisions, it’s essential to have visibility into how data is being used and whether it’s meeting the required quality standards. Data observability can help businesses identify patterns, gain insights, and make better decisions. It can also help to detect anomalies that might otherwise go unnoticed. With data observability, businesses can ensure that their data is reliable, accurate, and accessible, and that they can trust the insights they’re getting from it. Data integrity, however, is focused on more than data monitoring – it provides the ability to perform data validation and reconciliation in accordance with all types of data integrity concepts.
What is data integrity testing?
In the Gartner IT Glossary, data integrity testing is defined as verification that moved, copied, derived, and converted data is accurate and functions correctly within a single subsystem or application.
Data integrity processes should not only help you understand a project’s data integrity, but also help you gain and maintain the accuracy and consistency of data over its lifecycle. This includes data management best practices such as preventing data from being altered each time it is copied or moved. Processes should be established to maintain DWH/BI data integrity at all times.
Data integrity classifications for enterprise data environments
There are three primary types of data integrity:
- Entity integrity ensures that each row in a table, for example, is uniquely identified and without duplication. Entity integrity is often enforced by placing primary key and foreign key constraints on specific columns. Testing may be achieved by defining duplicate or the null values in test.
- Domain integrity requires that each set of data values/columns falls within a specific permissible defined range. Examples of domain integrity are correct data type, format, and data length; values must fall within the range defined for the system, null status, and permitted size values. Testing may be accomplished, in part, using null, default, and invalid values.
- Referential integrity is concerned with keeping the relationships between tables. Referential integrity is often enforced with primary key and foreign key relationships. It may be tested, for example, by deleting parent rows or child rows in tables.
The key capabilities of an enterprise data integrity solution
There’s a new type of data management solution to add to your tool kit that will enable you to impact the quality of your analytical, compliance, and operational data at scale. Tricentis Data Integrity brings the discipline of end-to-end, continuous, and automated testing solutions to the world of data, enabling you to catch more data errors upfront and prevent the costly downstream consequences of bad analytical and operational data. Data Integrity is a complementary solution to existing data management tools and works across enterprise application and analytics landscapes.
Conclusion
Data observability is a key component of any successful data-driven business. It ensures that data is accurate, reliable, and accessible, and that data quality issues are detected and resolved in real time. By investing in data observability, businesses can gain insight into how data is being used, identify patterns and anomalies, and make better decisions. Ultimately, data observability is a critical tool for organizations that want to remain competitive in today’s data-driven landscape.