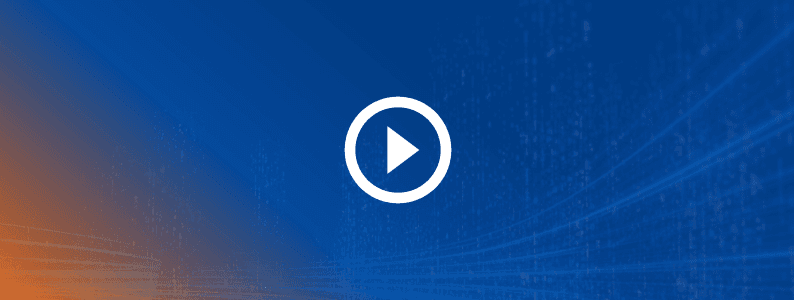
Agentic Test Automation in action
AI-powered testing assistant purpose-built for Tosca to enable...
Learn about the major threats to data integrity and threats to various ETL stages and how you can stop data deficiencies in their tracks.
Editor’s note: Wayne Yaddow is an independent consultant with over 20 years’ experience leading data migration/integration/ETL testing projects at organizations including J.P. Morgan Chase, Credit Suisse, Standard and Poor’s, AIG, Oppenheimer Funds, IBM, and Achieve3000. Additionally, Wayne has taught IIST (International Institute of Software Testing) courses on data warehouse, ETL, and data integration testing. He continues to lead numerous ETL testing and coaching projects on a consulting basis. You can contact him at wyaddow@gmail.com.
Data warehousing, integrations, and migrations are continually gaining importance as organizations attempt to transform the modern data explosion into insights that improve the customer experience and provide an edge against competition. However, data quality issues at various stages of ETLs are a major challenge to the rapid development and implementation of data integration solutions.
Many researchers have contributed to an understanding of data quality problems, collectively identifying general causes during these steps of data integration planning and execution:
The PDF that’s available below outlines the types of tests that this research found to identify data deficiencies across 8 data quality dimensions: accuracy, completeness, conformity, consistency, integrity, precision, timeliness, and uniqueness. I hope this information will help developers and others working on data integration solutions to expose and stop data deficiencies before moving to release.
Download the Data Testing Checklist PDF.
Learn more about how Tricentis automates data quality tests.
AI-powered testing assistant purpose-built for Tosca to enable...
We’re extending our proven expertise in test automation with...
Learn how to build a Workday testing strategy that minimizes...
Connect NeoLoad with Datadog to unlock real-time observability and...
Learn how a top European bank drove Agile transformation, tackled...