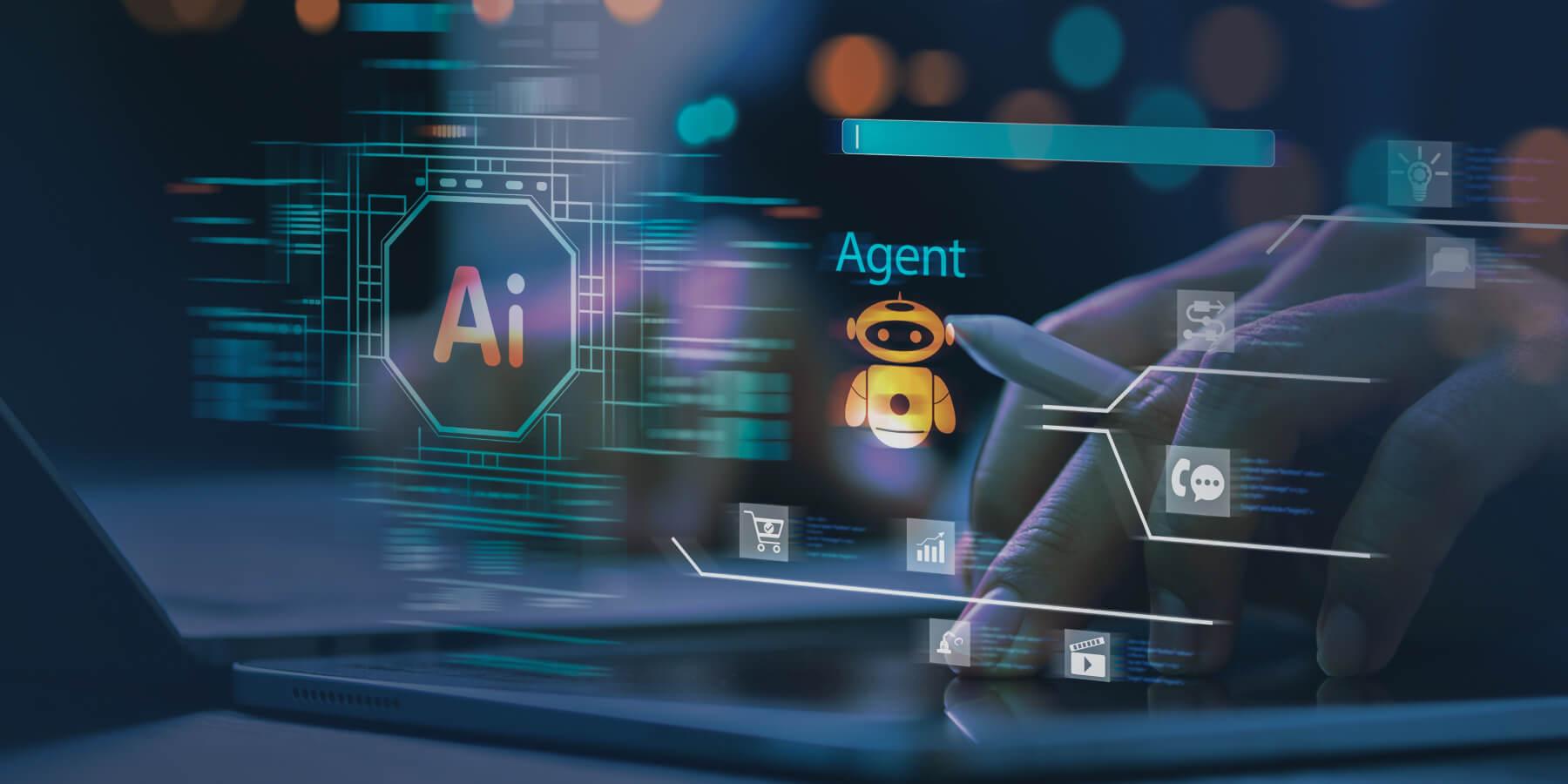
Tricentis unveils three major steps in AI-powered software testing
Introducing a powerful new way to coordinate and orchestrate complex workflows across AI agents and tools
Introducing a powerful new way to coordinate and orchestrate complex workflows across AI agents and tools
Learn how Cognitus leverages Tricentis to cut test cycle time by 60%, speed SAP data validation, and scale quality in complex SAP Cloud ERP transformation projects.
How Tricentis SeaLights helps catch AI logic bugs missed by traditional tests in agentic coding
A new executive order requires federal agencies to buy commercial software first to reduce costs and accelerate modernization.
Our Israel office recently hosted Junior Achievement students. Read how Tricentis invests in the next generations of innovators.
Bad data puts your Oracle environment at risk. Learn how to identify and minimize Oracle data quality issues and integrity gaps before they impact AI readiness.
Our key takeaways from SAP Sapphire Orlando 2025: Cloud migration and RISE with SAP, the SAP toolchain, and AI everything. See what we learned.
In many enterprise organizations, particularly across public sector and regulated industries, identity and access management (IAM) are not just a best practice; it’s a mandate.
We asked over 2,700 software delivery practitioners to understand what challenges they face with automated testing. Read the full report to learn more.
Top 5 challenges in continuous performance engineering—how NeoLoad ensures speed, scalability, and quality across delivery.
The 2025 Tricentis Global Partner Awards honor partners’ achievements in AI-powered quality engineering and innovation.
Collaboration across IT is increasingly critical to having a smooth and effective software release cadence. NeoLoad and Datadog can work together to help you deliver software with high quality that operates effectively.
In software development and QA, time matters. Discover how Testim’s new features can boost the efficiency of your testing workflows.
Discover how public sector teams automate Oracle testing to reduce risk, save time, and accelerate delivery without sacrificing compliance.
Organizations must prepare for increased accountability and standardization in data privacy, sovereignty, and ethical AI governance.
Oracle Cloud’s 25B release is coming in late April. Prepare your test plan to ensure new features don’t impact customizations or integrations.
This blog brings to light some of the “why” behind why we chose Anthropic’s Computer Using Agent, and what makes our agentic AI so powerful.
As federal agencies adapt to tighter budgets and smaller teams, they must find smarter ways to deliver secure, high-performing services.
Learn about new feature enhancements to the Vera platform with our 2025.1 release.
Tosca’s latest cloud release introduces advanced test design and authoring, zero-footprint execution, and more.
This release focuses on the addition of support for several new technologies, as well as the initial release of a fundamental modernization effort we have been implementing on the NeoLoad web platform.
This blog post explores a couple of reasons why your Salesforce implementation requires testing, and some best practices for how to optimize or implement a testing strategy.
In this blog, we explore the evolution, applications, training methodologies, and ethical considerations of LLMs summarized from our recent research published in the peer reviewed journal Computers, Materials and Continua.
The 2025 Oscars were meant to be a celebration of cinematic excellence, but for Hulu viewers, the biggest drama unfolded off-screen.